Breadcrumb
UICC 2019
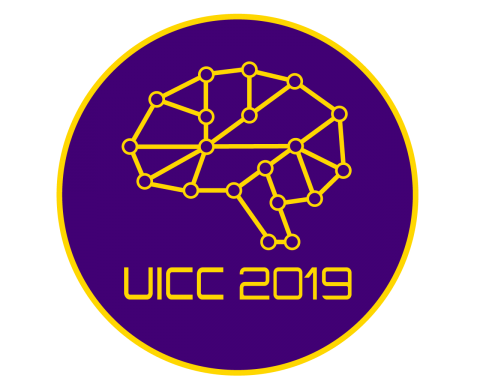
Schedule
Friday, February 22, 2019
5:00pm | Check-in; lobby area outside Shambaugh Auditorium, Main Library (LIB) |
5:30pm | Doors open; Shambaugh Auditorium, LIB |
6:00pm |
Welcome Address by Liam Crawford (ACM@UIowa President) and Professor Alberto Segre (UI Computer Science Chair); Shambaugh Auditorium, LIB Keynote Address by Sven Koenig (ACM Distinguished Speaker; University of Southern California) - Planning For Large Scale Robots; Shambaugh Auditorium, LIB |
7:30pm | Reception; Rotunda, Adler Journalism Building (AJB) |
8:30pm | Puzzle Competition, rules by ACM Officers; Rotunda, Adler Journalism Building (AJB) |
Saturday, February 23, 2019
8:30am | Continental breakfast and late check-in; Rotunda, AJB |
9:30am | Fahmida Hamid (Grinnell College) - Sentiment Analysis May Seem Deceptively Simple; 101 BCSB |
10:45am | Larry Birnbaum (Northwestern University) - From Contextual Search to Automatic Content Generation; 101 BCSB |
Noon | Lunch Break |
Noon | No-Tie Job Fair; Rotunda AJB (until 2pm) |
2:30pm | Tianbao Yang (University of Iowa) - Introduction to Learning with Big Data; 101 BCSB |
3:45pm | L. Miguel Encarnação (former Editor in Chief, IEEE Computer Graphics and Applications) - No Insight in Sight; 101 BCSB |
4:45pm | Door prize drawings, ACM Officers; 101 BCSB |
5:00pm | Pizza, subs and socializing with board games, card games; Rotunda, AJB |
Speakers
Keynote: Sven Koenig || Planning for Large-Scale Robots
Bio: Sven Koenig is a professor in computer science at the University of Southern California. Most of his research centers around techniques for decision making (planning and learning) that enable single situated agents (such as robots or decision-support systems) and teams of agents to act intelligently in their environments and exhibit goal-directed behavior in real-time, even if they have only incomplete knowledge of their environment, imperfect abilities to manipulate it, limited or noisy perception or insufficient reasoning speed. Additional information about Sven can be found on his web pages: idm-lab.org
Abstract: Multi-robot systems are now being used in industry. For example, hundreds of robots navigate autonomously in Amazon fulfillment centers to move inventory pods all the way from their storage locations to the inventory stations that need the products they store (and vice versa). Autonomous aircraft towing vehicles will soon tow aircraft all the way from the runways to their gates (and vice versa), thereby reducing pollution, energy consumption, congestion, and human workload. Path planning for these robots is difficult, yet one must find high-quality collision-free paths for them in real-time. Shorter paths result in higher throughput or lower operating costs (since fewer robots are required). I will discuss different versions of such multi-robot path-planning problems, algorithms for solving them, and their applications. I will also discuss a planning architecture that combines ideas from artificial intelligence and robotics. It makes use of a simple temporal network to post-process the output of a multi-robot path-planning algorithm in polynomial time to create a plan-execution schedule for robots that provides a guaranteed safety distance between them and exploits slack to absorb imperfect plan executions and avoid time-intensive re-planning in many cases.
Larry Birnbaum || From Contextual Search to Automatic Content Generation
Bio: Larry Birnbaum is Professor of Computer Science at Northwestern University, where he is Chair of the Computer Science Department and Co-Director of the Intelligent Information Laboratory, with a research focus on applied AI. He and his students build and research projects in natural language processing, intelligent information systems, social media data analytics, machine learning, computational journalism and media, conversational interfaces, and automatic content generation. Together with colleagues and students, Larry has published more than 140 papers on these topics, and holds 41 U.S. patents.
Larry received his BS and PhD degrees in Computer Science from Yale, and was on the faculty there before joining Northwestern.
Larry is also Co-Founder and Chief Scientific Advisor of Narrative Science, an AI start-up that builds and markets technology to automatically generate narratives from data, at scale. The company’s goal is simply this: To make the world’s data meaningful to people through stories.
Abstract: The astounding growth in data gathering, processing, storage, and networking capabilities over the past decades has opened the prospect of revolutionary advances in everything from medicine to media — if these data can actually be exploited properly. A key bottleneck is providing insight and understanding based on these data to people who need to make decisions using them. This talk will outline our work on contextual search aimed at helping people find information they need in the moment; on finding important and interesting patterns in data (especially social media); on the automation of editorial judgment; and on automatically generating stories based on those data to convey insights to people — and will include a number of demos of systems we’ve built. I’ll focus particularly on applications to journalism, and on the technology, originally developed at Northwestern, that has led to our start-up Narrative Science.
Tianbao Yang || Introduction to Learning with Big Data
Bio: Dr. Tianbao Yang received his Ph.D. degree in Computer Science from Michigan State University in 2012. Before joining UI, he was a researcher at NEC Laboratories America in Cupertino (2013-2014) and a Machine Learning Researcher at GE Global Research (2012-2013), mainly focusing on developing distributed optimization systems for various classification and regression problems. Dr. Yang has broad interests in machine learning and he has focused on several research topics, including large-scale optimization in machine learning, deep learning, and learning theory. His recent research interests revolve around accelerating convex optimization problems using error bound conditions. He has won the Best student paper award at 25th Conference on Learning Theory (COLT) in 2012. He is also an associate editor of the Neurocomputing Journal.
Abstract: In this talk, I will discuss fundamental issues of dealing with big data in machine learning, including why big data is important, what are the challenges in solving big data problems, and how to optimize different machine learning problems with big data. I will also talk about our recent research on large-scale stochastic optimization for optimizing deep neural networks and generative adversarial nets.
Fahmida Hamid || Sentiment Analysis May Seem Deceptively Simple: Look for Cues but Be Careful
Bio: Dr. Fahmida Hamid is an Assistant Professor at Grinnell College in Grinnell, Iowa. Professor Hamid received her Ph.D. from The University of North Texas in 2016. Her major areas of interest are Information Retrieval and Natural Language Processing. Her work includes modeling an unsupervised sentiment-oriented summary extraction technique from a single document, proposing a generic baseline for evaluating automatic summary (and keyphrase) extraction algorithms, and devising a relativized scale for evaluating machine-generated summaries with respect to multiple reference summaries.
Currently, she is researching keyphrase extraction from scientific articles, topic-based sentiment lexicon generation, and design of augmented question-answering systems.
Abstract: Information, especially user-generated contents, is a good mixture of facts and sentiments. Sentiments are no less important than facts in many ways. At personal level: we like to hear public opinions before buying a new car, watching a new movie, or planning a family trip to a new place; at organizational level: democratic governments may avoid upcoming conflicts if they analyze the public sentiments on a rising issue before it is too late. With the advent of Web technology, posting of personal views/opinions has become easy. On the other hand, monitoring, managing, and extracting useful information from the user-generated contents can be intimidating for anyone (person or organization). Detecting, extracting, and classifying opinions and sentiments from texts, known as "Sentiment Analysis", is one of the popular recent topics among Natural Language Processing (NLP) researchers.
A recent study shows that 20% of online sales are directly impacted by public sentiments, highlighting the importance of the applications of Sentiment Analysis. But analyzing sentiments can be challenging for several reasons. User-generated contents do not always follow standard formats, bloggers may express their opinions implicitly in long forum posts, or they may just post emoticons or short-phrased tweets. In summary, Sentiment Analysis is an interesting but difficult task. This talk will discuss some applications, common approaches, challenges, and future research directions in Sentiment Analysis.
L. Miguel Encarnação || No Insight in Sight – Data Visualization and its Challenges in a World of Big Data
Bio: Dr. L. Miguel Encarnação has been a cross-industry strategic R&D and innovation leader for over two decades, specializing technologies for creating insight from data to drive improved decision-making and innovation. He recently accepted the position as Professor of Practice at the University of Iowa as a dual appointment with the Tippie College for Business in Management Sciences and the College of Arts and Sciences in Computer Science. Prior leadership positions in R&D, innovation, and workforce development include roles as SVP and Chief innovation Officer at ACT, Inc. in Iowa City, IA, Chief Technology and Innovation Officer at the QED Group in Arlington, VA, Director for Emerging Technology Innovation at Humana in Louisville Kentucky, and President and CTO at The IMEDIA Academy in Providence, RI. Dr. Encarnação is the former editor-in-chief of the IEEE Computer Graphics and Applications magazine, and the author of numerous contributions to peer-reviewed journals and conferences. He holds BS, MS, and PhD degrees in Computer Science with an emphasis on Human-Computer Interaction, Virtual and Augmented Reality, Data Visualization, Machine Learning, and AI.
Abstract: Data Visualization has established itself as an important discipline in supporting various stakeholders throughout the data analytics process. Modern tools and techniques for scientific and information visualization allow the human user to actively participate in shaping, cleaning, modeling, analyzing, and communicating data, and to control the related decision-making processes. However, the mere size, velocity, and diversity of Big Data poses new challenges to established data visualization concepts and techniques, so that even rethinking of their roles in the analytical process seems worth considering. This talk will discuss visualization in the context of Big Data, highlight some of its challenges and identify some recent research and development in the emerging field of Big Data Visualization.